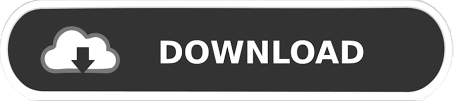
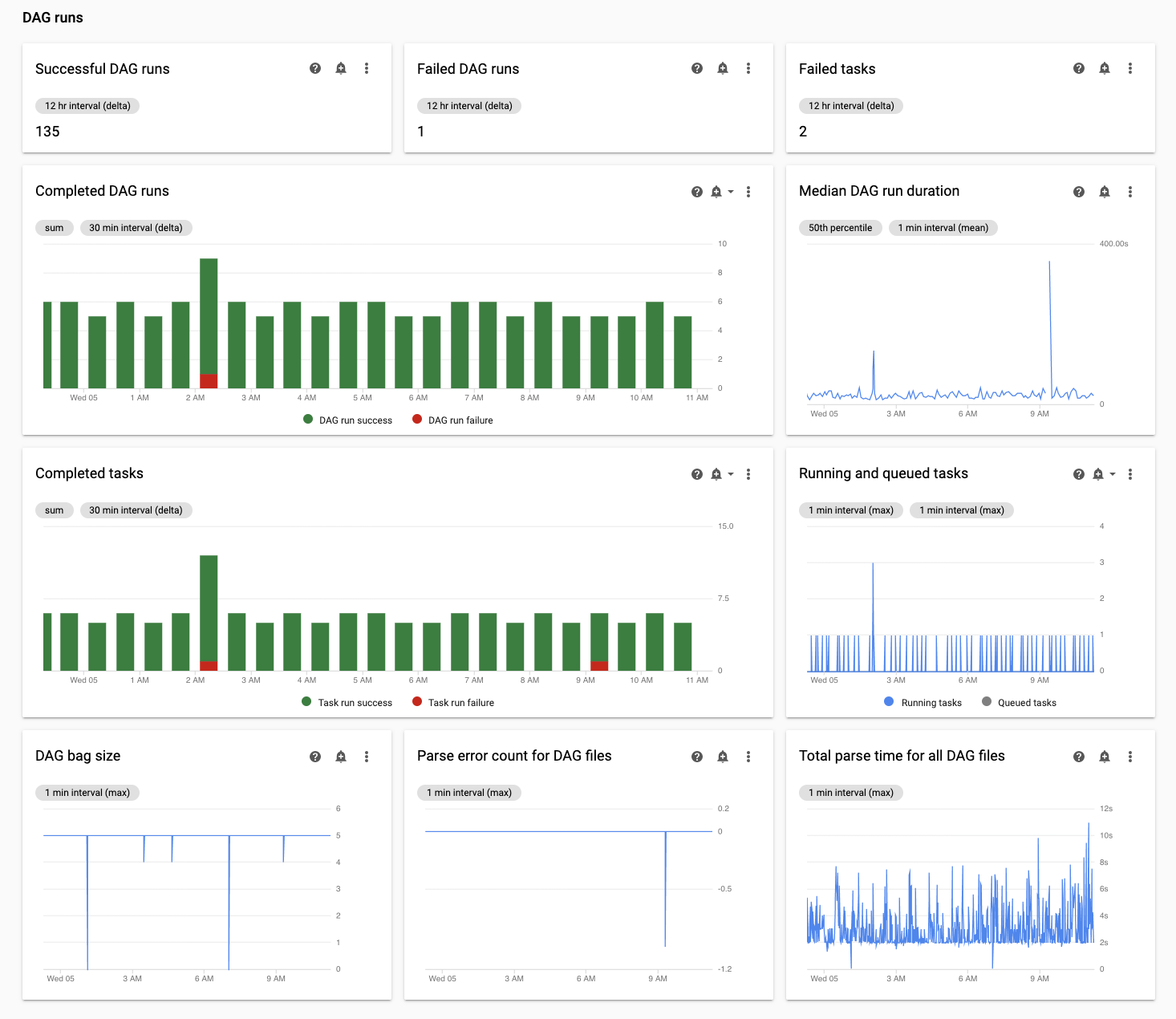
Note: If you're looking for documentation for master branch (latest development branch): you can find it on s./airflow-docs.įor more information on Airflow's Roadmap or Airflow Improvement Proposals (AIPs), visit the Airflow Wiki. Visit the official Airflow website documentation (latest stable release) for help with installing Airflow, getting started, or walking through a more complete tutorial.
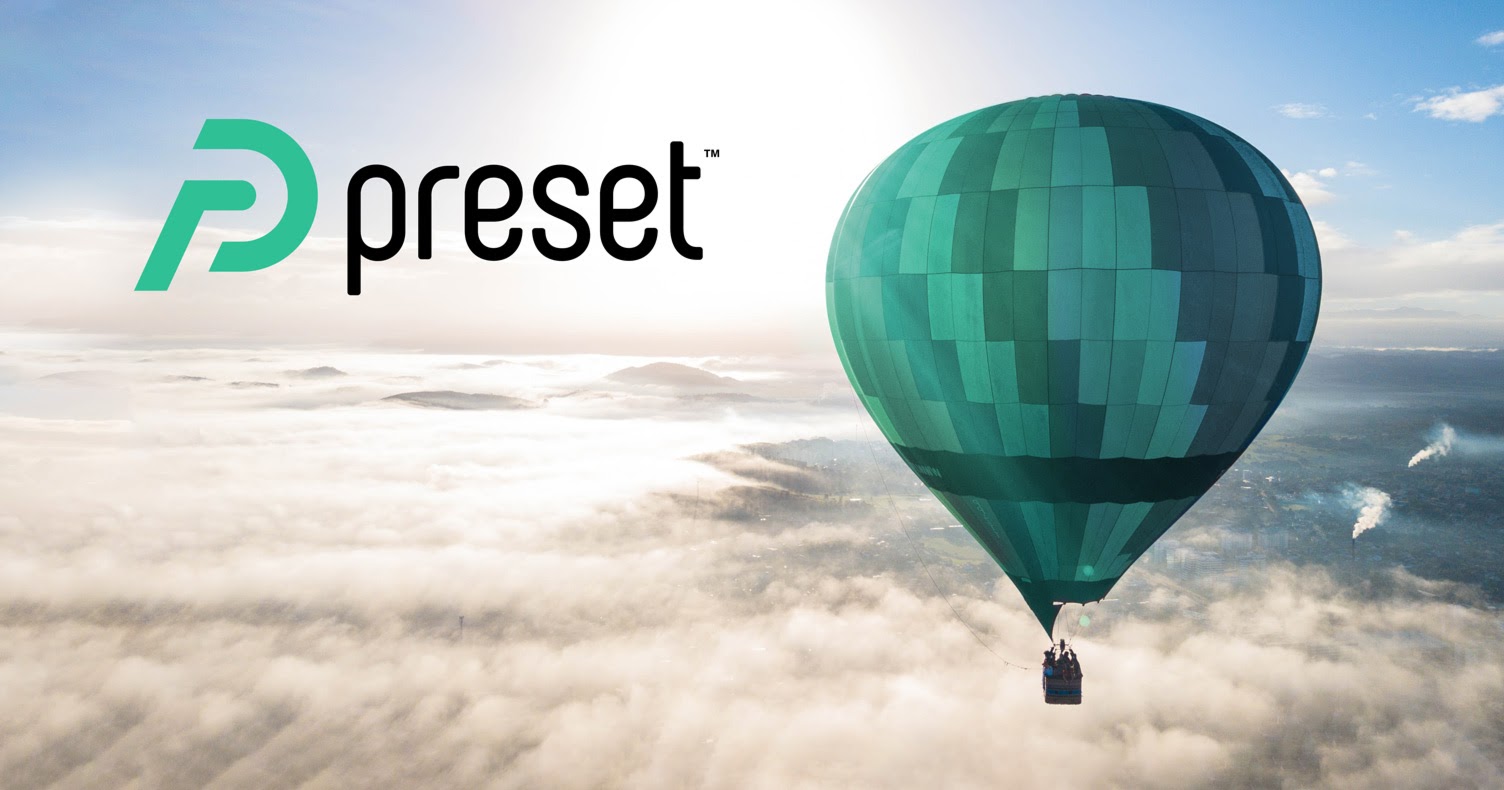
Additional notes on Python version requirements Running multiple schedulers - please see the "Scheduler" docs. Note: MariaDB and MySQL 5.x are unable to or have limitations with
#Apache airflow documentation code#
This allows for writing code that instantiates pipelines dynamically. Dynamic: Airflow pipelines are configuration as code (Python), allowing for dynamic pipeline generation.For high-volume, data-intensive tasks, a best practice is to delegate to external services that specialize on that type of work.Īirflow is not a streaming solution, but it is often used to process real-time data, pulling data off streams in batches. results of the task will be the same, and will not create duplicated data in a destination system), and should not pass large quantities of data from one task to the next (though tasks can pass metadata using Airflow's Xcom feature).
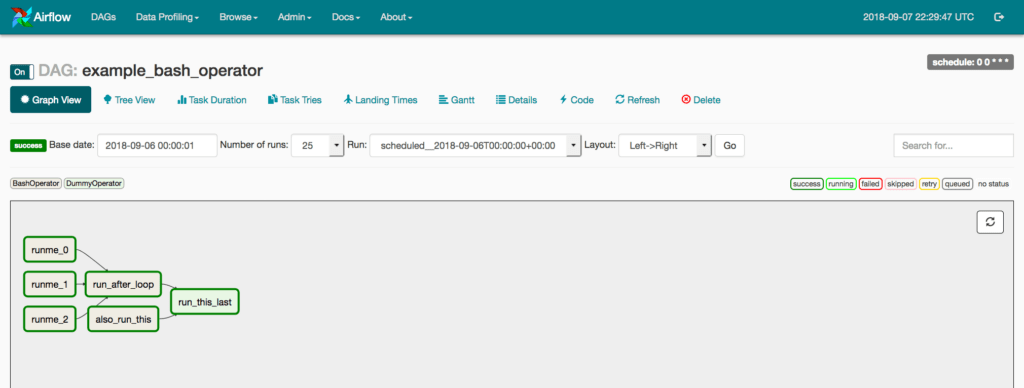
Other similar projects include Luigi, Oozie and Azkaban.Īirflow is commonly used to process data, but has the opinion that tasks should ideally be idempotent (i.e. When DAG structure is similar from one run to the next, it allows for clarity around unit of work and continuity. Can I use the Apache Airflow logo in my presentation?Īirflow works best with workflows that are mostly static and slowly changing.The rich user interface makes it easy to visualize pipelines running in production, monitor progress, and troubleshoot issues when needed. Rich command line utilities make performing complex surgeries on DAGs a snap. The Airflow scheduler executes your tasks on an array of workers while following the specified dependencies. Use Airflow to author workflows as directed acyclic graphs (DAGs) of tasks. When workflows are defined as code, they become more maintainable, versionable, testable, and collaborative. Users can create their own functions to do special-purpose processing.Īpache Pig is released under the Apache 2.0 License.Apache Airflow (or simply Airflow) is a platform to programmatically author, schedule, and monitor workflows. The way in which tasks are encoded permits the system to optimize their execution automatically, allowing the user to focus on semantics rather than efficiency. Complex tasks comprised of multiple interrelated data transformations are explicitly encoded as data flow sequences, making them easy to write, understand, and maintain. It is trivial to achieve parallel execution of simple, "embarrassingly parallel" data analysis tasks. Pig's language layer currently consists of a textual language called Pig Latin, which has the following key properties: The salient property of Pig programs is that their structure is amenable to substantial parallelization, which in turns enables them to handle very large data sets.Īt the present time, Pig's infrastructure layer consists of a compiler that produces sequences of Map-Reduce programs, for which large-scale parallel implementations already exist (e.g., the Hadoop subproject). Apache Pig is a platform for analyzing large data sets that consists of a high-level language for expressing data analysis programs, coupled with infrastructure for evaluating these programs.
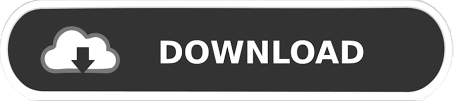